The artificial intelligence (AI) stack refers to the technologies, frameworks, libraries, and tools used to develop and function AI applications. There is a fusion of several layers or components to enable tech AI stack capabilities.
Here are some major AI Stacks consisting of the following layers and components:
AI Stack Layers
1. Data layer
This data layer includes collections, storage, and management of databases that are mandated for training and testing AI models.
2. Machine Learning Layer
This machine-learning layer includes algorithms, models, and data to predict and make informed decisions based on learning.
3. Deep Learning Layer
This functions as a subset of machine learning, including artificial neural networkings by permitting a sizable amount of the database.
4. Natural Language Processing (NLP) layer
This layer in AI uses algorithms and models that help in processing to understand human inputs and their language.
5. Computer Vision Layer
This layer includes the use of algorithms to analyze and interpret visual information from images and videos.
6. Robotics Layer
This robotics layer ensures the right physical mechanism of AI technologies by controlling and automating
7. AI Infrastructure Layer
This layer consists of hardware, software, and cloud services that require building, training, and deploying AI models and regular applications.
AI Stack Components
The AI stack contains a few particular components of this technology, and their applications and use cases can occasionally change.
Here are some common components are:
1. Data storage and management
This component consists of a long database and helps in data management by organizing and storing large records in AI applications. Such as SQL and NoSQL databases, Hadoop, and Spark.
2. Data Preprocessing and Feature Engineering
This component allows cleaning and data processing for AI usage. It identifies relevant features to train models, and tools to access components. It is used in components like Python’s Panda library, apache, spark, and the sci-kit learn.
3. Machine Learning Algorithms
This component of the AI tech stack supervises machine learning by building predictive modeling. It includes linear regression, decision trees, k-means clustering, and neural networking.
4. Deep Learning Frameworks
This component mentions about framework enabling the training and deployment of learning models, and neural networks with many layers. For example, TensorFlow, PyTorch, and Keras.
5. Natural Language Processing (NLP) Tools
This component involves tools that are used to process, analyze, and generate human sentiments and understanding for an AI. Its examples are NLTK, spaCy, and GPT-3.
6. Computer Vision Tools
This component analyzes the whole process and data in visuals, video recognition, object detection, and segmentation. It has great examples available are OpenCV, TensorFlow Object Detection API, and YOLO.
7. Robotics Tools
This component consists of a tool for creating and operating robots that use AI principles, such as computer vision and learning capabilities.
8. Cloud Infrastructure
This component comprises cloud-based services providing scalable computing power and AI application storage. Examples are Amazon Web Services (AWS), Google Cloud Platform, and Microsoft Azure.
What are Artificial Intelligence Stack Technologies?
Here are some common stack technologies that are applicable in the Artificial intelligence (AI) stack:
1. Python
Python is among the popular programming languages used for generating AI applications. It allows accessibility over a wide range of libraries, and frameworks for data processing and machine learning.
2. R Programming
R is also another popular programming language implemented in AI applications, for statistical modeling and data analysis.
3.TensorFlow
It is an open-source framework for generating machine training and building strong learning modules. It allows support to various applications, including computer potential and natural processing.
4. PyTorch
PyTorch is also one open-source framework for building and training deep learning models. It allows a more dynamic approach toward model building than TensorFlow, making experiments more easy and impactful.
5. Keras
Keras is proficient in neural network APIS. It is useful in both TensorFlow and PyTorch. It offers a simple interface helping to build training deep learning models, making it easy for beginners to start with AI.
6.Scikit-Learn
It is a well-recognized machine-learning library for Python. It allows diverse algorithms for classification, regression, clustering, and dimensional reduction. Using this preprocessing and model selection becomes easy.
7. Apache Spark
It is a distributed computing framework, used for processing large datasets. It allows support for data processing, machine learning, and graph processing.
8. OpenCV
OpenCV is an open-source computer vision library used to detect and analyze images, videos, object detection, and facial recognition.
9. Natural Language Toolkit (NLTK)
NLTK is a Python library language processing. It enables tools to access tokenization, part-of-speech tagging, named entity recognition, and sentiment analysis.
10. Amazon Web Services (AWS)
AWS is a cloud computing platform that offers a wide array of services for building AI applications. Some examples include Amazon Sagemaker, AWS Deep Learning AMIs, and Amazon Recognition.
AI Stack includes diverse technologies depending on specific applications and their usage. They work together to build intelligent applications learning from data and making predictions.
AI Technology Stack Application
Artificial intelligence works uniquely based on specific applications and usage. Here are some common ways to use AI stack:
1. Data Preparation
In general AI stack starts with data collection, preparation, and where data can easily be processed means of using AI models. It involves data extraction using various sources, databases, APIs, and sensors.
2. Model Development
Model AI stack is enabling the development of machine learning or deep learning models. It includes a selection of appropriate algorithms, training models, and performance evaluation.
3. Deployment
This AI stack involves packaging and its dependencies into a container. The deployment process includes setting up infrastructure for scaling and model monitoring.
4. Inference
This AI stack is used to make predictions or to take decisions on new data. This process involves inference of passing data through the model generation.
5. A feedback loop
This AI stack includes a feedback loop where the output of the model is used to demonstrate updation or improvement in modeling. This helps in collecting performance, analysis, and improvement suggestions in the model.
Modern AI Stack
The Modern AI stack includes a blend of source tools, cloud services, and specialized hardware for building and deploying AI applications.
Here are some major components to include in the modern AI stack.
1. Data storage and management
The first and foremost step in building an AI application is data collection, storage, and management. It includes databases, data lakes, cloud storage, or Google Cloud storage.
2. Data processing
The next step involves processing making it perfect for AI models. It includes data cleaning, normalization, feature extraction, and others. It includes data cleaning, data normalization, or feature extraction.
3. Machine learning frameworks
After the second step, this machine-learning framework helps in data processing. These frameworks generally include TensorFlow, PyTorch, and Scikit-learn.
4. Deep learning frameworks
This framework includes specialized forms of learning particularly in complex data such as images, video, and text.
5. Model serving and deployment
This model and deployment include production environments. It includes AWS SageMaker, Google AI platform, or Microsoft Azure Machine Learning.
6. Specialized Hardware
This stack is used to accelerate model training and inference. It improves the demand for AI applications and specialized hardware such as GPUs or TPUs.
AI stack is a complex, fast, and rapidly evolving ecosystem of AI tools and technologies. It enables the development and deployment of smart applications.
Artificial Intelligence Stack Tools
Data Storage & Management
- Databases (e.g. PostgreSQL, MySQL)
- Data Lakes (e.g. AWS S3, Azure Data Lake)
- Cloud Storage (e.g. AWS S3, Google Cloud Storage)
Data Processing
- Apache Spark
- Apache Flink
- Apache Kafka
Machine Learning Frameworks
- TensorFlow
- PyTorch
- Sci-kit-learn
Deep Learning Frameworks
- TensorFlow
- Keras
- PyTorch
Model Serving & Deployment
- AWS SageMaker
- Google AI Platform
- Microsoft Azure Machine Learning
- Kubeflow
- Seldon
- MLflow
Model Monitoring and Management
- Prometheus
- Grafana
- Kibana
Specialized Hardware
- GPUs
- TPUs
Conclusion
Today’s stack AI offers outstanding benefits in several areas, helping to meet the rising need for technological innovation. There are numerous tools for creating an AI stack as well as outstanding technology that make challenges easier. If you’re searching for a reputable AI consulting company, you can visit buildfuture, they will meet all you AI development services needs.
FAQs
1: What is AI Stack?
In layman’s terms, the artificial intelligence stack refers to the collection of technologies, frameworks, libraries, and tools that enable the building and deployment of AI applications.
2: What are the unique components of the AI tech stack?
The AI tech stack runs on a conceptual component model. It consists of a data layer, a machine learning layer, a deep learning layer, a computer vision layer, a robotics layer, an AI infrastructure layer, and Natural language processing.
3: How does the tech AI stack protect security and privacy?
AI tech stacks protect users’ security and privacy by enabling behavior modeling, identifying malware, and automating measures to counter user attacks.
4: Which technologies are commonly used in the AI tech stack?
To handle the largest database efficiently, the AI tech stack uses data processing technologies like Apache Spark and Apache Hadoop. Using these technologies, AI improves data visualization and exploration capabilities.
5: Which cloud platforms are commonly integrated into AI tech stacks?
AI tech stacks utilize cloud platforms to make data processing, and storage more viable. It includes Amazon Web Services (AWS), Microsoft Azure, or Google Cloud Platform (GCP).
6: What are some commonly used Machine learning frameworks used in AI?
Tech AI uses machine learning based on mathematical algorithms and statistics. The most widely used Tech AI frameworks include TensorFlow, PyTorch, Scikit-Learn, Spark ML, Torch, and Keras.
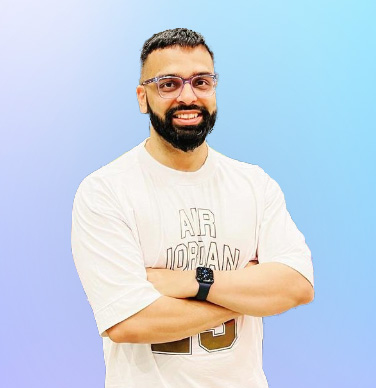
I am the CEO and founder of Blocktech Brew, a team of blockchain and Web 3.0 experts who are helping businesses adopt, implement and integrate blockchain solutions to achieve business excellence. Having successfully delivered 1000+ projects to clients across 150+ countries, our team is dedicated to designing and developing smart solutions to scale your business growth. We are focused on harnessing the power of Web 3.0 technologies to offer world-class blockchain, NFT, Metaverse, Defi, and Crypto development services to businesses to help them achieve their goals.